Data fuels enterprise AI value: 6 takeaways from the Gartner Hype Cycle for Artificial Intelligence, 2023
GenAI may be the most transformative technology of the past decade but data is where enterprises are able to realize real value from AI today.
GenAI may be the most transformative technology of the past decade, according to analysts in the newly published Gartner® Hype Cycle™ for Artificial Intelligence, 2023.
The report paints a picture of a technology poised to transform the enterprise AI landscape. But, while genAI warrants excitement, data will provide the key to unlocking its transformative value.
Data is your differentiator. Models have become larger, more powerful, more available, and more commoditized. Data remains proprietary.
“Data quality, curation, and consistency often improve AI accuracy more efficiently than tweaking models,” the report said.
The Gartner® Hype Cycle™ reports offer a graphic representation of the maturity and adoption of technologies and applications, and how they are potentially relevant to solving real business problems and exploiting new opportunities.
This year’s report also recognized Snorkel AI as a Sample Vendor for both data-centric AI as well as data labeling and data annotation.
Below follows six of our favorite takeaways from this report, with a focus on how data will continue to play a fundamental role in both genAI and traditional machine learning workflows.
AI remains high-value or transformative for enterprises
Compared with other sectors Gartner® investigates, AI promises to yield the greatest value, the research firm said.
“The AI Hype Cycle has more innovations with benefit ratings in the high to transformational categories, with no innovation having a benefit rating of low or moderate,” the report said.
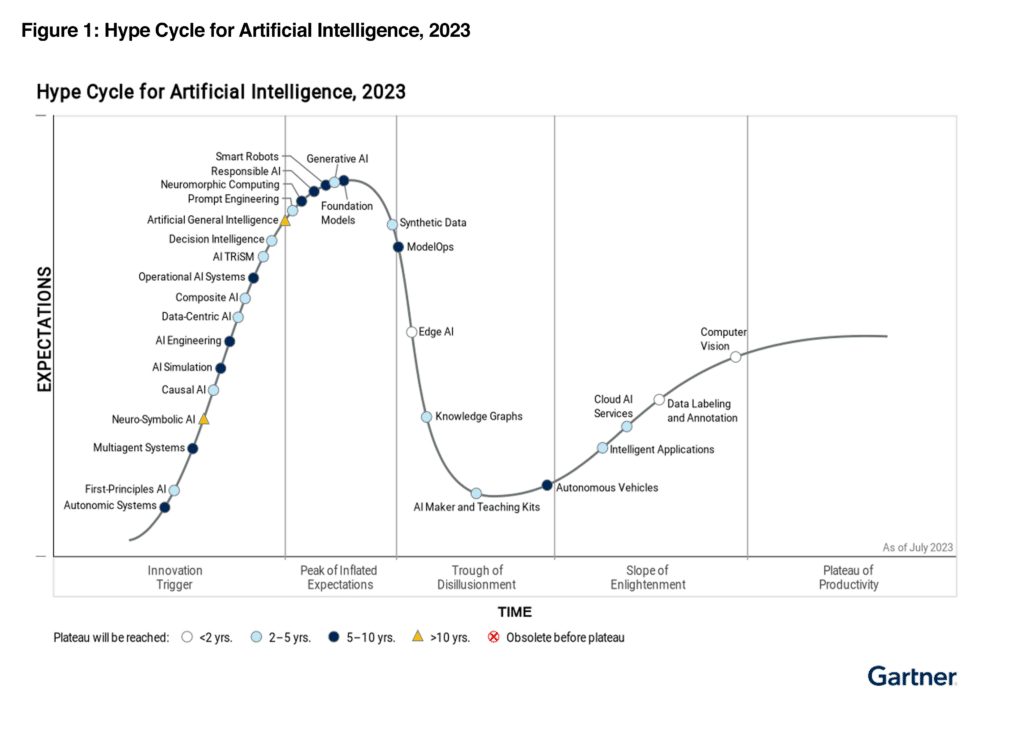
GenAI likely at maximum gap between potential and usage
While Gartner® said that genAI “has had an impact like no other technology in the past decade,” the firm also said that “there is still a large gap between the expected potential impact and actual usage.”
Considering the report placed genAI at the peak of the Hype Cycle™, that gap is likely at (or near) the biggest it will ever be.
Our own investigations have found that enterprises are eager to use GenAI in production, but face serious challenges in doing so. Firms aiming to close that gap will need data-centric approaches as well as data labeling and annotation to do so.
Data and Data labeling and annotation essential to genAI value
“The need for better training data has increased to remove the bottleneck in developing AI solutions—especially those particular to generative AI and industry use cases.” —Gartner® Hype Cycle™ for Artificial Intelligence, 2023
We agree with Gartner on this Data is the programming language for AI and machine learning. And better data builds better models.
Snorkel researchers recently demonstrated this principle in collaboration with Together AI. The team labeled the original fine-tuning dataset for the RedPajama large language model according to task type and quality and then strategically curated the corpus. The resulting model performed better than its parent model, according to human testers in a double-blind experiment.
Crowd-labeling services present challenges for enterprises
Third-party labeling workers present challenges in terms of both label quality and information security, the report noted.
“Especially for those DL&A services that bring in public crowds, many clients feel uneasy distributing certain data to virtually unknown parties,” the report said.
Last year’s incident in which outsourced data labelers shared sensitive images on social media may have reinforced those concerns.
While reputation and prequalification systems have emerged to address customer doubts, quality and security concerns persist.
Data-centric approaches vital to genAI future
Gartner rated data-centric AI approaches as “embryonic,” with 5% or less of the target audience using them. The report also noted that data-centric AI is “becoming especially important with the rise of pretrained off-the-shelf models.”
Data-centric practices demonstrate their most obvious importance at the time of model training. But Nurtekin Savas, head of enterprise data science at Capital One told the audience at our Future of Data-Centric AI virtual conference that data-centric methods can (and should) extend across the entire data lifecycle.
Obtaining and labeling real-world data presents a real burden
The burden involved in “obtaining real-world data and labeling it” currently presents a “major” challenge in getting value out of AI, the report said.
While synthetic data can help—and sometimes stands as the only reasonable solution—it is not a cure-all. As the report noted elsewhere, simulations cannot fully replicate real-world systems. Real-world data, curated, managed, maintained, and labeled through data-centric approaches and enriched with expert domain knowledge, will usually yield better-performing models.
Final thoughts: labeled data as gasoline
The Gartner® Hype Cycle™ for Artificial Intelligence 2023 report presents genAI as the machine of the future. But machines require fuel. Many have called data the new oil. Labeled data, then, is the new gasoline: refined and ready to power the machine forward.
See our deeper dive into the report’s findings here, or get your complimentary copy of the Gartner® Hype Cycle™ for Artificial Intelligence 2023 report here.
Hype Cycle™ for Artificial Intelligence, 2023
GARTNER® is a registered trademark and service mark of Gartner® and Hype Cycle™ are a registered trademark of Gartner, Inc. and/or its affiliates in the U.S. and internationally and are used herein with permission. All rights reserved. Gartner® does not endorse any vendor, product or service depicted in its research publications, and does not advise technology users to select only those vendors with the highest ratings or other designation. Gartner® research publications consist of the opinions of Gartner’s research organization and should not be construed as statements of fact. Gartner® disclaims all warranties, expressed or implied, with respect to this research, including any warranties of merchantability or fitness for a particular purpose.
Matt Casey leads content production at Snorkel AI. In prior roles, Matt built machine learning models and data pipelines as a data scientist. As a journalist, he produced written and audio content for outlets including The Boston Globe and NPR affiliates.