Create domain-specific LLM evaluations
Move beyond “vibe checks” by adding domain- and task-specific LLM evaluations which provide far more granular and insightful metrics than general, off-the-shelf benchmarks, and take into account unique business policies and standards. An enterprise LLM evaluation framework must assess model performance against relevant criteria.
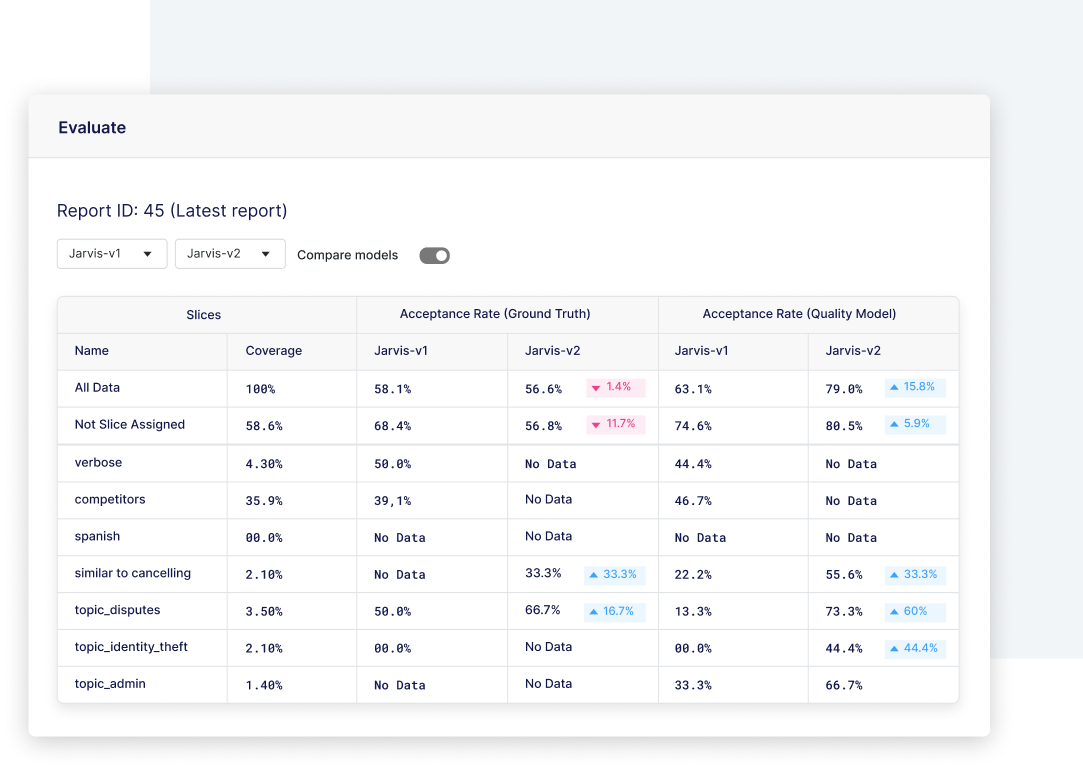
How can SME insights scale LLM evaluations?
Snorkel Flow provides data scientists and SMEs with the ability to define business- and domain-specific acceptance criteria for LLM responses. Rather than requiring SMEs to manually review every response, Snorkel Flow uses their acceptance criteria to train a quality model. The quality model then acts as a proxy for SMEs, scaling their knowledge to predict whether LLM responses will be accepted or rejected – like a human evaluation process but faster and scalable.
LLM evaluation for enterprise AI applications
Improve evaluation speed, accuracy, and consistency
Tailor LLM evaluations to domain-specific tasks
Adapt to new insights and evolving requirements
Easily modify LLM evaluation criteria at any time and redefine how prompts are “sliced” into different categories, allowing for the evaluation of LLM accuracy on new axes and as business policies, standards, and requirements continue to evolve. As the LLM system advances, the evaluation framework evolves alongside it, seamlessly adapting to new insights and simplifying the assessment of model performance over time.
Iterate on models faster, and deploy with confidence
Quickly and consistently evaluate LLM accuracy based on business- and domain-specific criteria to understand the strengths and weaknesses of different models, whether or not model accuracy has improved after further training and identify areas where additional training data is required. This robust LLM evaluation system provides quick feedback, allowing for faster adjustments to models, shorter training cycles and more reliable LLMs.
Dive deeper into LLM evaluation with these resources
Deploy specialized AI to production today with Snorkel
Snorkel Flow
Snorkel Custom
Our team of experts will fast-track specialized model development on your data to reduce model development costs, accelerate time to production, and achieve higher model quality.