Future of Data-Centric AI day 1: LLMs changed the world
Snorkel AI hosted the first day of the two-day The Future of Data-Centric AI virtual conference today, featuring Matei Zaharia, the creator of Spark, DJ Patil, the first U.S. Chief Data Scientist, and other esteemed speakers and panelists.
Across thirteen sessions, the speakers commented on the current and future state of artificial intelligence and machine learning. They focussed largely on the challenges and opportunities in leveraging large language models and foundation models, as well as data-centric AI development approaches.
Below follows a summary of the day’s highlights, along with a full rundown of all other sessions.
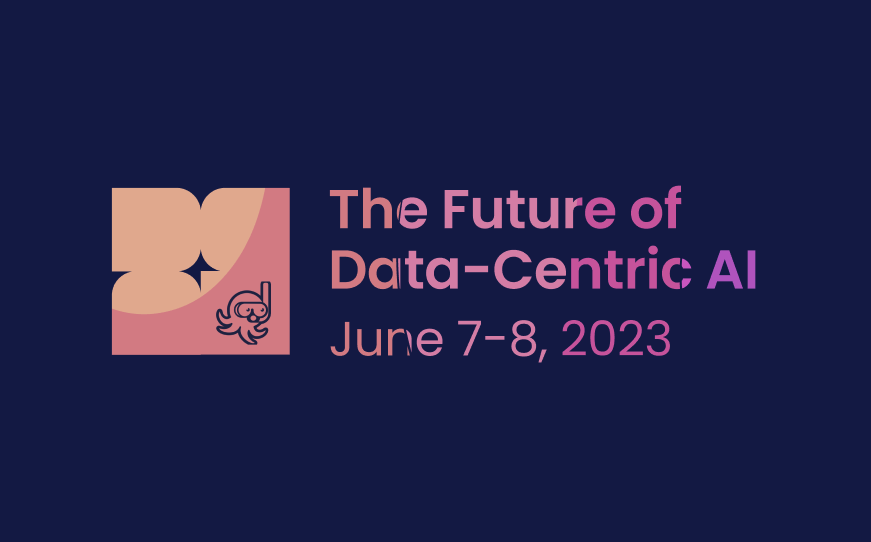
Top Highlights
Bridging the Last Mile: Applying Foundation Models with Data-Centric AI
Alex Ratner, CEO and co-founder of Snorkel AI, set the tone for the day in his opening keynote address. The session highlighted the “last mile” problem in AI applications and emphasized the importance of data-centric approaches in achieving production-level accuracy.
Foundation models, Alex said, yield results that are “nothing short of breathtaking,” but they’re not a complete answer for enterprises who aim to solve challenges using machine learning.
“We are, in our view, in a bit of a hype cycle,” he said. Enterprises and data science teams, he said, are excited to work with these new technologies, but coaxing from them the performance necessary for a production application takes a lot of additional work.
In response to audience questions, Alex said companies should invest in data teams oriented around domain knowledge and data-centric methods. He also said that data scientists should not worry about optimizing themselves out of a job. The net “boom” in data-centric jobs, he said, would be “orders of magnitude” greater than the number of jobs foundation models optimize away.
Fireside chat: Alex Ratner and Gideon Mann on building BloombergGPT
In the second fireside chat, Gideon Mann, Head of Machine Learning product and Research in the office of the CTO at Bloomberg LP, talked with Alex about how his organization built the first major specialized enterprise GPT model, BloombergGPT.
Mann touched on many details of the project—from the mix of the data Bloomberg used to their training sequence to the impact of the numerical precision of gradient estimates to the challenge in evaluating the performance of generative AI models.
Mann’s formal education in machine learning, he said, came during a time when you optimized a model against a test set. The output of generative models defies simple comparisons to test sets.
Alex and Mann agreed that the shape of computer programming would change, but that it would not be going away. While foundation models have a lot of promising capabilities, they work better as part of an ecosystem—one that involves data curation and additional resources—which means programmers and data scientists still have plenty to do.
Fireside chat: The role of data in building Stable Diffusion and Generative AI
Emad Mostaque, founder and CEO of Stability AI, talked with Ratner about the stunning progress made in the field of artificial intelligence and foundation models and how the use of these models might progress in the near future.
The new abundance of different sizes of foundation models with different specializations allows machine learning practitioners to approach problems in new ways. Instead of having one big model, programmers will be able to network together several models in different architectures.
“You probably need an AI to glue them all together, to be honest,” Mostaque said.
Alex noted that the current paradigm could also result in “family trees” of models, where smaller cheaper models are derived from larger ones.
Data-Driven Government: A Fireside Chat with the Former U.S. Chief Data Scientist
In the third and last of the day’s fireside chats, Alex and former U.S. chief data scientist DJ Patil held a wide-ranging conversation covering topics including the value of regulation and the origin and value of the term “data scientist.”
The term, Patil said, sprang from an exercise in which he and others posted job descriptions for what we would now call data science roles, but under a variety of job titles. Applicants applied to the “data science” posting more often than any of the others, he said, so that became the standardized title.
“It is actually ambiguous,” Patil said. “People don’t actually know what it means. So that prevents you from being put in a box.”
The pair agreed on the value of the title—noting that it empowered data scientists to focus on a problem and then devise a solution without being anchored to a particular discipline or set of tools.
Patil also said he wants the US to invest more in artificial intelligence.
“We are not investing enough. This is a Sputnik moment. This is a moment of Go,” Patil said. “We need to be treating this as a national effort to go all in on what this is.”
Poster Competition
In a first for FDCAI, we ran a live research poster competition. The competition began with 15 posters on the FDCAI Slack workspace, which we narrowed down to three finalists who presented during the day’s sessions.
In a tight vote, University of Cambridge PhD student Nabeel Seedat won the top prize of a GPU workstation from Lambda worth approximately $8,000. His project, Data-IQ, won the audience’s approval with its promise of allowing users to profile their training data with just two lines of code.
Columbia University Ph.D. student Zachary Huang took the second-place prize of $5,000 dollars worth of cloud GPU credits from Lamba with a presentation on JoinBoost, a package he created that allows users to train tree models inside databases faster and more safely than traditional methods.
Rutgers Universit Ph.D. Honglu Zhou took home the third place prize, $2,000 in cloud GPU credits for a presentation about a method she pioneered to allow foundation models to understand instructional videos.
LLMOps: Making LLM Applications Production-Grade
Matei Zaharia, co-founder and chief technologist at Databricks, discussed techniques for transforming large language models into reliable, production-grade applications. In particular, he highlighted his company’s Demonstrate-Search-Predict framework which abstracts away aspects of using foundation models, such as prompt engineering.
Full session recap
Panel – The Linux Moment of AI: Open-Sourced AI Stack
The panel, moderated by MLOps Community founder Demetrios Brinkmann, featured experts from Seldon, Predibase, and Hugging Face. They discussed the impact of open-source models and tools on revolutionizing AI. Open-source foundation models are getting better and smaller thanks to data-centric approaches, they noted, and that’s changing how businesses approach machine learning.
A Practical Guide to Data-Centric AI – A Conversational AI Use Case
Daniel Lieb, senior director of model risk management at Ally Financial, and Samira Shaikh, director of data science at the same company, showed how their organization is using data-centric approaches, generative AI and LLMs to set up a conversational AI for Ally Auto customers.
Panel – Adopting AI: With Power Comes Responsibility
Harvard’s Vijay Janapa Reddi, JPMorgan Chase & Co.’s Daniel Wu, and Snorkel AI’s Aarti Bagul explored the ethical challenges of leveraging generative AI in the midst of an ML arms race. They touched on AI regulation, risk mitigation strategies, and the importance of data-centric ML systems in ensuring responsible AI innovation.
The Future is Neurosymbolic
Echoing themes from elsewhere in the conference, AI21 Labs Co-Founder and Co-CEO Yoav Shoham noted that the current generation of foundation models are “seductive,” but imperfect.
“If you’re brilliant 90% of the time and nonsensical or just wrong 10% of the time, that’s a non-starter,” he said. “It kills all credibility or trust, and you can’t have that.”
Generating Synthetic Tabular Data That’s Differentially Private
Gretel AI Senior Applied Scientist Lipika Ramaswamy surveyed the privacy limitations of current generative models and the reactive nature of thwarting adversarial attacks. She explored the application of differential privacy as a solution—including a specific approach that combines measuring low dimensional distributions and learning a graphical model representation.
Fireside Chat: The Building Blocks of Modern Enterprise AI
Aparna Lakshmi Ratan from Snorkel AI and Marco Casalaina from Azure Cognitive Services delved into the core elements of modern enterprise AI, exploring the convergence of data, models, and MLops platforms. They discussed the impact of model form factors, data types, use cases, enterprise constraints, and the use of private data in shaping the AI landscape.
Panel: Navigating the LLM Labyrinth in a World of Rules
Chris Booth from NatWest Group, Nadia Wood from the Mayo Clinic, and Harshini Jayaram from Snorkel AI discussed the complexities of using large language models in regulated industries, focusing on strategies for reducing errors and misinterpretations in conversational AI applications.
Catch the sessions you missed!
The Future of Data-Centric AI 2023, our two-day free virtual conference, brought together thousands of data scientists, AI/ML practitioners, researchers, and the AI community at large to hear about and discuss the latest trends and research in data-centric AI. If you registered for the event but didn't see all the sessions you wanted, you can now catch up. The recorded sessions are available for registrants at the same Zoom portal as the live sessions.
Matt Casey leads content production at Snorkel AI. In prior roles, Matt built machine learning models and data pipelines as a data scientist. As a journalist, he produced written and audio content for outlets including The Boston Globe and NPR affiliates.