On-demand webinar
Speakers

Shane Johnson
Senior Director of Product Marketing
Snorkel AI
I started out as a developer and architect before pivoting to product/marketing. I'm still a developer at heart (and love coding for fun), but I love advocating for innovative products -- particularly to developers.
I've spent most of my time in the database space, but lately I've been going down the LLM rabbit hole.
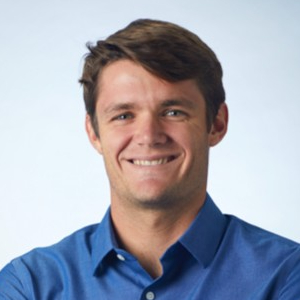
Charles Dickens
Applied Research Scientist
Snorkel AI
My general research interests are in machine learning, modeling richly structured graph data, and enhancing artificial intelligence systems with symbolic reasoning capabilities. I have developed models for a variety of applications, including computer vision, language modeling, social networks, demand forecasting, and recommender systems. I am currently seeking a research or applied scientist role where I can contribute my skills to produce impactful research and products.
Improving the accuracy of domain-specific tasks with LLM distillation
In this webinar, we provide an overview of LLM distillation, explain how it compares with fine-tuning, and introduce the latest techniques for training SLMs using foundation models and knowledge transfer methods.