Search result for:
Events & Conferences
Join us at an upcoming industry event or conference
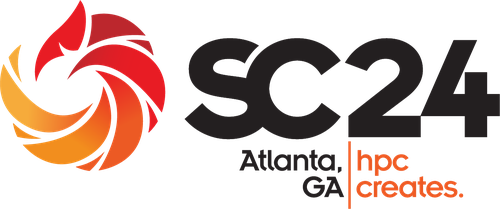
SuperComputing HPC show
November 19, 2024 | 10 AM
Atlanta, GA
High Performance Computing, Networking, Storage, and Analysis
View conference
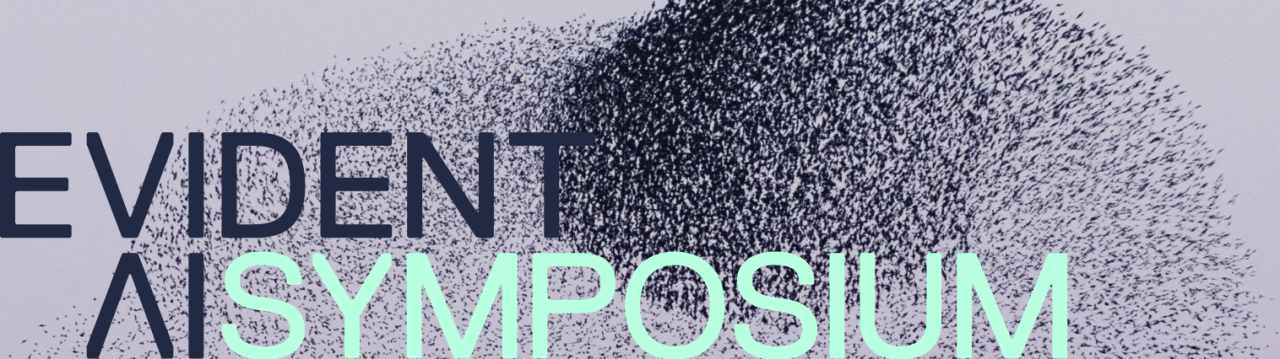
Evident AI Symposium
November 21, 2024 | 10 AM
New York, NY
In partnership with Bloomberg
View event
AWS Re:Invent
December 2 - 6, 2024 | 10 AM
Las Vegas NV
A learning conference hosted by AWS for the global cloud-computing community
View conference
AI Summit NY
December 11 - 12, 2024 | 10 AM
New York, NY
The AI Summit New York celebrates its ninth year
View event
Generative AI Summit London
March 31 - April 2, 2025 | 10 AM
London
The second annual event.
View event
Databricks: Data + AI Summit
June 9 - 12, 2025 | 10 AM
San Francisco, CA
Annual conference hosted by a leading provider of data analytics and AI platforms.
View conference